Understanding Data Analytics in Business
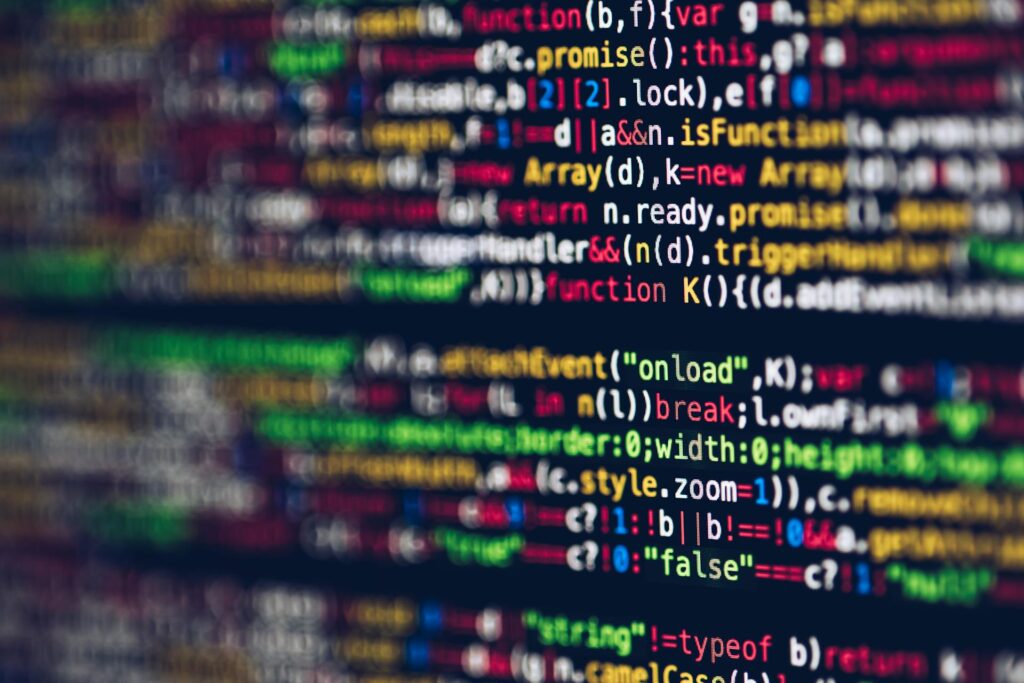
In today’s digital age, data has become the lifeblood of businesses across industries. The ability to collect, process, and derive insights from vast amounts of information is revolutionizing how companies operate and make decisions. Data analytics refers to the process of examining raw data to uncover patterns, draw conclusions, and support business decisions.
At its core, data analytics involves using specialized systems and software to transform large volumes of raw data into meaningful insights that can guide strategy and operations. Some key components of effective data analysis include:
- Data collection: Gathering relevant data from various sources, including customer interactions, sales transactions, website traffic, social media, IoT devices, etc.
- Data processing: Cleaning, organizing, and structuring raw data to make it suitable for analysis. This often involves removing errors, filling in missing values, and formatting data consistently.
- Data analysis: Applying statistical techniques and analytical models to identify trends, correlations, and patterns in the processed data. This could involve descriptive, diagnostic, predictive, or prescriptive analytics depending on the business needs.
- Data interpretation: Translating analytical findings into actionable business insights that can inform decision-making. This requires both technical skills and business acumen.
- Data visualization: Presenting data and insights visually through charts, graphs, dashboards etc. to make them easily understandable for stakeholders.
The importance of data analytics in business has grown exponentially in recent years. As the volume of data generated continues to skyrocket, companies that can effectively harness this information gain a significant competitive advantage. Data-driven insights enable businesses to:
- Make more informed and accurate decisions
- Identify new opportunities and revenue streams
- Optimize operations and reduce costs
- Enhance customer experiences through personalization
- Mitigate risks and detect fraud
- Develop innovative products and services
The evolution of data analytics in the corporate world has been remarkable. In the past, businesses relied primarily on historical reporting and basic statistical analysis. Today, advanced analytics powered by artificial intelligence and machine learning can process massive datasets in real-time to generate predictive insights and prescriptive recommendations.
Cloud computing and big data technologies have made sophisticated analytics accessible to organizations of all sizes. User-friendly visualization tools have also democratized data analysis, allowing non-technical business users to explore data and generate insights.
As data becomes increasingly central to business success, companies are investing heavily in building robust data infrastructure and analytics capabilities. The role of data scientists and analysts has become pivotal, with these professionals working closely with business leaders to drive data-informed decision making across the organization.
The Role of Data Analytics in Decision-Making
Data analytics is transforming business decision-making by providing leaders with evidence-based insights to guide their choices. Rather than relying solely on intuition or past experiences, executives can now leverage data to make more informed and strategic decisions.
Some key ways data analytics supports decision-making include:
Turning raw data into actionable insights: Analytics tools can process vast amounts of structured and unstructured data to uncover meaningful patterns and trends. For example, a retailer might analyze point-of-sale data, inventory records, and customer profiles to optimize pricing and promotions. The insights generated can directly inform pricing decisions to maximize revenue.
Enhancing strategic planning: Predictive analytics allows businesses to forecast future trends and outcomes based on historical data. This empowers leaders to develop more robust strategic plans. For instance, a manufacturer could use predictive models to anticipate demand fluctuations and adjust production capacity accordingly.
Identifying market opportunities: By analyzing market data, customer behavior, and competitor information, companies can spot untapped opportunities. A financial services firm might use analytics to identify underserved customer segments or geographies ripe for expansion.
Testing hypotheses and validating assumptions: Data analytics enables businesses to test different scenarios and validate hypotheses before committing resources. For example, an e-commerce company could use A/B testing to evaluate the impact of website design changes on conversion rates.
Supporting real-time decision-making: Modern analytics platforms can process data streams in real-time, allowing for rapid insights and decision-making. This is particularly valuable in fast-moving industries like stock trading or online advertising.
Reducing human bias: Data-driven insights can help counteract cognitive biases that often creep into human decision-making. By basing choices on objective data rather than gut feelings, businesses can avoid common pitfalls.
Quantifying uncertainty and risk: Advanced analytics techniques like Monte Carlo simulations can help leaders better understand and quantify the risks associated with different decisions.
To illustrate how data analytics drives decision-making in practice, consider a large retail chain looking to optimize its store locations. The company could analyze demographic data, foot traffic patterns, competitor locations, and historical sales data to determine the most promising sites for new stores. Predictive models could forecast expected revenue for different locations, allowing executives to make data-informed decisions on where to expand.
Similarly, a B2B software company might leverage customer usage data, support tickets, and renewal rates to identify accounts at risk of churning. This insight could trigger proactive interventions by the customer success team to improve retention.
While data analytics provides powerful decision support, it’s important to note that it should complement rather than replace human judgment. The most effective approach combines analytical insights with domain expertise and business acumen.
Benefits of Incorporating Data Analytics
Integrating data analytics into business processes can yield substantial benefits across various aspects of operations. Some key advantages include:
Improving operational efficiency: Analytics can uncover inefficiencies and bottlenecks in business processes, enabling organizations to streamline operations. For example:
- A logistics company might use route optimization algorithms to reduce fuel costs and delivery times.
- A manufacturer could analyze production data to identify and eliminate causes of defects or downtime.
- An HR department could use workforce analytics to improve employee scheduling and resource allocation.
Enhancing customer experience: Data analytics allows businesses to gain deeper insights into customer behavior and preferences, enabling more personalized experiences. Benefits include:
- Tailored product recommendations based on purchase history and browsing patterns.
- Customized marketing messages and offers for different customer segments.
- Proactive customer service interventions based on predictive models.
- Improved product development informed by customer feedback and usage data.
Driving innovation and product development: Analytics can guide innovation efforts by revealing unmet customer needs and market gaps. For instance:
- A consumer goods company might analyze social media sentiment to identify emerging product trends.
- An automotive manufacturer could use IoT data from connected cars to inform new feature development.
- A pharmaceutical firm might leverage genomic data analysis to accelerate drug discovery.
Gaining competitive advantage: Organizations that effectively leverage data analytics can outperform competitors in several ways:
- More accurate demand forecasting leading to better inventory management.
- Dynamic pricing strategies that maximize revenue.
- Targeted marketing campaigns with higher ROI.
- Faster time-to-market for new products and services.
Improving risk management: Advanced analytics techniques can help businesses better identify, assess, and mitigate various risks:
- Fraud detection algorithms can flag suspicious transactions in real-time.
- Predictive maintenance models can prevent costly equipment failures.
- Supply chain analytics can highlight potential disruptions before they occur.
Enhancing financial performance: Data-driven insights can directly impact a company’s bottom line through:
- Increased revenue from improved customer targeting and retention.
- Cost savings from optimized operations and resource allocation.
- Better cash flow management through accurate forecasting.
To illustrate these benefits, consider a large e-commerce platform that implemented advanced analytics across its operations. By analyzing customer browsing and purchase data, the company was able to create personalized product recommendations, leading to a 25% increase in average order value. Predictive analytics models optimized inventory levels, reducing stockouts by 30% while decreasing warehousing costs. Machine learning algorithms improved fraud detection, cutting losses by millions annually.
Implementing Data Analytics Strategies
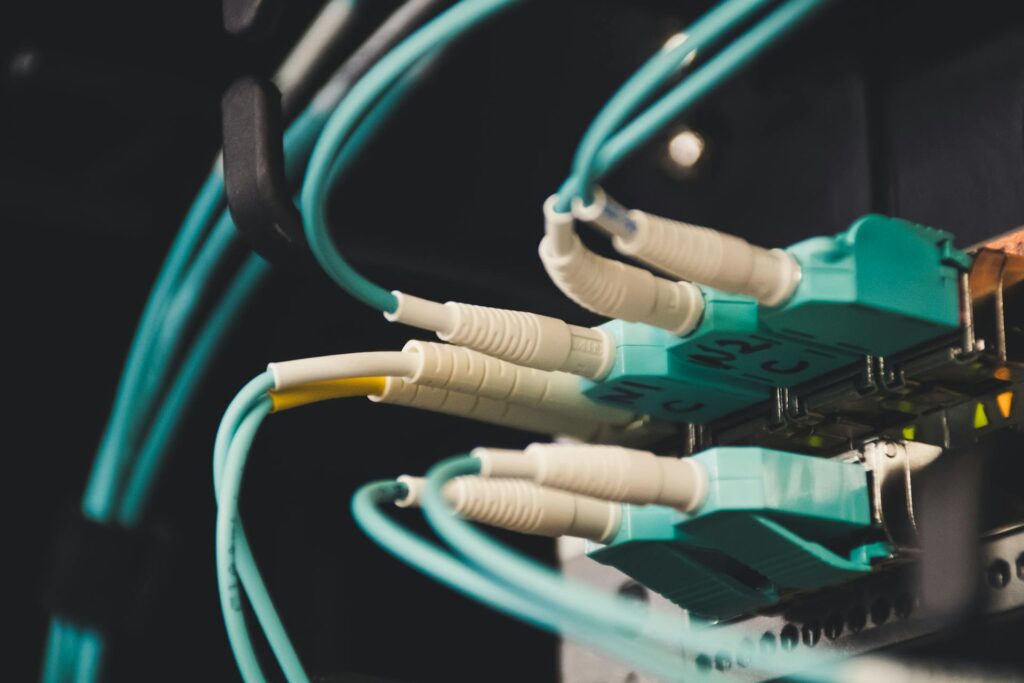
Successfully implementing data analytics requires a strategic approach that aligns with business objectives. Key steps include:
1. Choosing the right analytical tools and technologies:
- Assess your organization’s specific needs and data maturity level.
- Consider factors like scalability, ease of use, integration capabilities, and total cost of ownership.
- Popular options include cloud-based platforms (e.g., AWS, Google Cloud), business intelligence tools (e.g., Tableau, Power BI), and open-source frameworks (e.g., Python, R).
2. Building a skilled data analytics team:
- Hire or train professionals with expertise in statistics, data science, and domain knowledge.
- Key roles may include data engineers, data analysts, data scientists, and visualization specialists.
- Foster a culture of continuous learning to keep up with evolving technologies and techniques.
3. Integrating data analysis into business processes:
- Identify key decision points where data-driven insights can add value.
- Develop standardized processes for data collection, analysis, and reporting.
- Ensure analytics outputs are easily accessible to relevant stakeholders.
- Implement feedback loops to continuously refine and improve analytical models.
4. Fostering a data-driven culture:
- Secure buy-in from leadership and communicate the value of data analytics across the organization.
- Provide training to improve data literacy among non-technical employees.
- Encourage data-informed decision making at all levels of the company.
- Celebrate and share success stories to reinforce the benefits of analytics.
5. Ensuring data quality and governance:
- Implement robust data management practices to ensure accuracy, consistency, and security.
- Develop clear policies around data access, usage, and privacy compliance.
- Regularly audit and clean data to maintain its integrity.
6. Starting with high-impact, achievable projects:
- Begin with well-defined use cases that can demonstrate quick wins.
- Focus on projects that align closely with key business objectives.
- Use initial successes to build momentum and secure further investment.
A phased approach often works well when implementing data analytics strategies. For example, a mid-sized retailer might start by focusing on basic descriptive analytics to gain insights into sales trends and customer segmentation. As the organization builds capabilities and sees results, it could progress to more advanced predictive and prescriptive analytics projects.
Challenges and Solutions in Data Analytics
While data analytics offers immense potential, organizations often face several challenges in implementation:
1. Data quality issues:
- Challenge: Inaccurate, incomplete, or inconsistent data can lead to flawed insights.
- Solution: Implement robust data governance practices, including data cleansing, validation, and regular audits. Invest in data quality tools and establish clear data ownership.
2. Data privacy and security concerns:
- Challenge: Stricter regulations (e.g., GDPR) and growing privacy concerns complicate data usage.
- Solution: Develop comprehensive data privacy policies, implement strong security measures, and ensure compliance with relevant regulations. Use anonymization and encryption techniques where appropriate.
3. Lack of skilled personnel:
- Challenge: There’s a shortage of professionals with advanced data analytics skills.
- Solution: Invest in training existing staff, partner with universities, consider outsourcing or using managed analytics services. Leverage user-friendly analytics tools to empower business users.
4. Integration of disparate data sources:
- Challenge: Data often resides in silos across different systems and departments.
- Solution: Implement a centralized data warehouse or data lake. Use ETL (Extract, Transform, Load) tools to consolidate data from various sources.
5. Resistance to change:
- Challenge: Employees may resist adopting data-driven approaches.
- Solution: Foster a data-driven culture through leadership endorsement, training programs, and showcasing early wins. Involve end-users in the analytics process.
6. Scalability and performance issues:
- Challenge: As data volumes grow, traditional systems may struggle to process information efficiently.
- Solution: Leverage cloud-based analytics platforms, implement distributed computing frameworks (e.g., Hadoop), and optimize data storage and processing techniques.
7. Interpreting complex analytical outputs:
- Challenge: Non-technical stakeholders may struggle to understand and act on sophisticated analyses.
- Solution: Focus on clear data visualization and storytelling. Provide training on interpreting analytical results. Create user-friendly dashboards and reports.
8. Balancing speed and accuracy:
- Challenge: There’s often pressure to deliver quick insights, which can compromise thoroughness.
- Solution: Implement agile analytics practices, use rapid prototyping, and clearly communicate the trade-offs between speed and accuracy to stakeholders.
By proactively addressing these challenges, organizations can maximize the value they derive from their data analytics initiatives.
Unlocking the Transformative Power: Real-World Examples
To truly understand the impact of data analytics on business decision-making, it’s helpful to examine real-world examples across different industries:
1. Retail: Boosting sales through customer insights
A large clothing retailer struggled with inventory management and personalized marketing. By implementing advanced analytics, they were able to:
- Analyze purchase history, browsing behavior, and demographic data to create detailed customer segments.
- Use predictive models to forecast demand for different products by region and season.
- Develop personalized product recommendations, increasing online conversion rates by 35%.
- Optimize inventory allocation across stores, reducing stockouts by 20% and markdowns by 15%.
- Create targeted marketing campaigns, improving email open rates by 50% and click-through rates by 30%.
The result was a 10% increase in overall sales and a 5% improvement in profit margins within the first year.
2. Manufacturing: Streamlining operations and improving quality
A global automotive manufacturer leveraged data analytics to enhance its production processes:
- Implemented IoT sensors across the production line to collect real-time data on machine performance.
- Used predictive maintenance models to identify potential equipment failures before they occurred, reducing unplanned downtime by 30%.
- Analyzed quality control data to identify factors contributing to defects, leading to a 25% reduction in defect rates.
- Optimized supply chain operations by analyzing supplier performance, weather patterns, and transportation data, cutting lead times by 15%.
- Improved product design by analyzing warranty claims and customer feedback data.
These initiatives resulted in annual cost savings of over $100 million and significantly improved product quality ratings.
3. Financial Services: Enhancing risk management and fraud detection
A large bank implemented advanced analytics to improve its risk assessment and fraud detection capabilities:
- Developed machine learning models to analyze transaction patterns and flag potentially fraudulent activities in real-time.
- Created more accurate credit risk models by incorporating alternative data sources like social media activity and mobile phone usage.
- Used natural language processing to analyze customer service interactions and identify early warning signs of dissatisfaction or potential defaults.
- Implemented network analysis techniques to uncover complex fraud rings and money laundering schemes.
These efforts led to a 40% reduction in fraud losses, a 20% improvement in loan default predictions, and enhanced regulatory compliance.
4. Healthcare: Improving patient outcomes and operational efficiency
A hospital network used data analytics to enhance patient care and streamline operations:
- Analyzed patient records, treatment outcomes, and genetic data to develop personalized treatment plans.
- Used predictive models to identify patients at high risk of readmission, enabling targeted interventions.
- Optimized staff scheduling by analyzing patient flow patterns and historical demand data.
- Implemented a real-time bed management system to reduce wait times and improve resource allocation.
These initiatives resulted in a 15% reduction in average length of stay, a 25% decrease in readmission rates, and significant improvements in patient satisfaction scores.
5. E-commerce: Personalizing customer experiences and optimizing pricing
A leading online marketplace leveraged data analytics to enhance its platform:
- Developed sophisticated recommendation engines using collaborative filtering and machine learning algorithms.
- Implemented dynamic pricing models that adjust in real-time based on demand, competitor pricing, and other factors.
- Used A/B testing to optimize website design and user experience across different devices.
- Analyzed customer service interactions to identify common pain points and improve self-service options.
These efforts led to a 20% increase in average order value, a 30% improvement in customer retention rates, and a 10% boost in overall revenue.
These examples illustrate how data analytics can drive transformative changes across various industries and business functions. By leveraging data-driven insights, organizations can make more informed decisions, optimize operations, and gain a significant competitive advantage in today’s fast-paced business environment.
Embracing the Data-Driven Future
As we’ve explored throughout this article, data analytics is revolutionizing the way businesses operate and make decisions. The ability to extract meaningful insights from vast amounts of data is becoming a critical differentiator in today’s competitive landscape.
To fully embrace the data-driven future, organizations should:
- Invest in data infrastructure: Build robust systems for collecting, storing, and processing data at scale.
- Develop analytics capabilities: Hire skilled professionals and provide ongoing training to build a strong analytics team.
- Foster a data-driven culture: Encourage data-informed decision-making at all levels of the organization.
- Prioritize data quality and governance: Implement strong practices to ensure data accuracy, security, and compliance.
- Start small and scale: Begin with high-impact projects and gradually expand analytics initiatives across the organization.
- Stay agile: Be prepared to adapt analytics strategies as technologies evolve and new opportunities emerge.
- Balance automation with human insight: While leveraging advanced analytics, remember the importance of domain expertise and human judgment.
- Focus on actionable insights: Ensure that analytics efforts translate into tangible business value and improved decision-making.
As we look to the future, emerging technologies like artificial intelligence, machine learning, and the Internet of Things will further amplify the power of data analytics. Organizations that successfully harness these capabilities will be well-positioned to thrive in an increasingly data-driven world.
By embracing data analytics and fostering a culture of continuous learning and improvement, businesses can unlock new levels of efficiency, innovation, and competitive advantage. The transformative power of data-driven decision-making is only beginning to be realized, and the organizations that adapt quickly will be the ones that shape the future of their industries.